Congratulations to Prof. Zhi Pei and his Ph.D. students Haimin Lu for Paper Acceptance
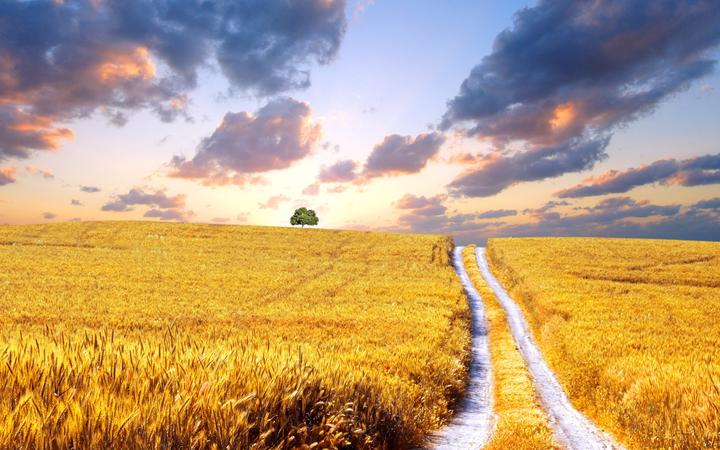
Congratulations to Prof. Zhi Pei and his Ph.D. students Haimin Lu for their paper was accepted by the European Journal of Operational Research.
Title
Target-based Distributionally Robust Optimization for Single Machine Scheduling
Abstract
In the traditional single-machine scheduling problem with uncertain processing time, distributionally robust optimization models are established in situations where the distribution information is not known exactly. One well-studied robust optimization method used in scheduling is the distributionally β-robust optimization technique. The goal of this technique is to maximize the probability that the total flow time of the job sequence does not exceed the predetermined target level T. However, it is possible that the excess value of the total flow time beyond the target T may be incredibly large, and therefore it is necessary to consider the optimized excess value. To address this concern, two new target-based risk measures are introduced. First, target-oriented CVaR, the worst-case conditional value-at-risk (CVaR) is used to minimize the excess value beyond T, which accounts for both the excess probability and the excess value. Second, the essential riskiness index (ERI) is extended to a multi-piecewise linear function for excess value control in single-machine scheduling problems. To obtain a tractable closed-form solutions of the risk measures, the CVaR-based measure is decomposed into an assignment problem and a second-order cone programming problem, and the ERI is converted to its equivalent semi-definite programming and second-order cone counterparts. To efficiently solve the risk measures for single-machine scheduling, a bisection search algorithm and a Benders decomposition algorithm are designed. In the numerical analysis, the excess value and the probability are investigated with respect to two distributions, namely the two-point distribution and the truncated normal distribution. The experiment design also considers different ranges of expectation and variance, tolerance levels, and problem scales. It is discovered that the CVaR and ERI-based methods are always better than the distributionally β-robust scheduling approach in terms of excess value.